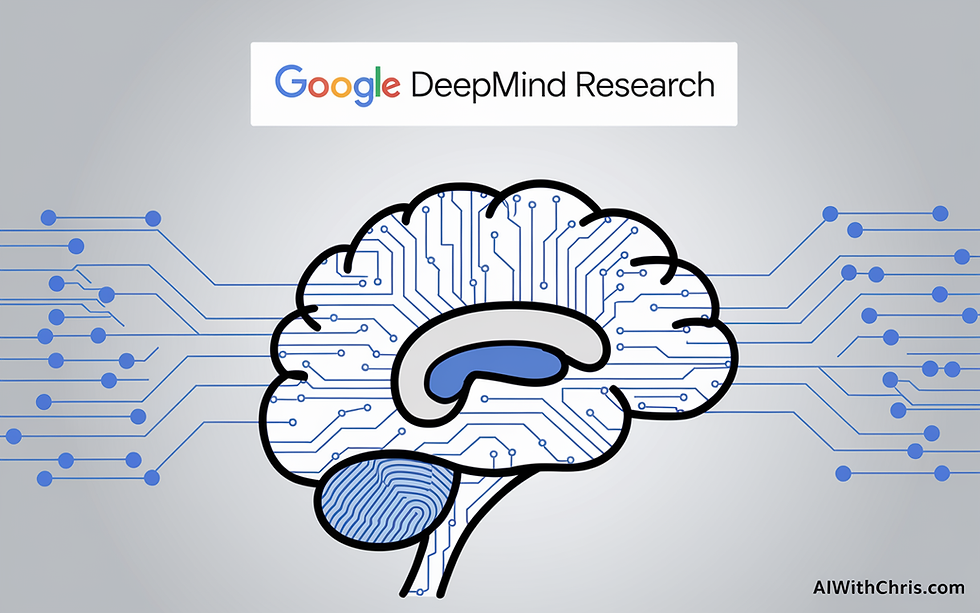
Understanding LLMs and Their Importance in Regression Analysis
Large Language Models (LLMs) have become a focal point in artificial intelligence research due to their remarkable ability to understand and generate human language. However, their potential extends beyond mere language generation, impacting various fields, such as regression analysis. Regression analysis is a statistical method used for predicting continuous outcomes based on input variables.
This technique is vital across numerous domains, including finance, healthcare, and social sciences. Google's DeepMind has been at the forefront of exploring the capabilities of LLM embeddings to enhance regression techniques, providing insights into how these advanced models can extract and utilize valuable features from data.
DeepMind's Innovative Approach to Embeddings
DeepMind researchers have made significant advances in using LLM embeddings to represent complex data in simpler forms that are easier for regression models to process. The embeddings act as condensed representations of high-dimensional data, effectively capturing relationships that traditional methods may overlook.
By leveraging these embeddings, regressions can be performed on nuanced data sets with improved accuracy and interpretability. This innovative approach provides a blueprint for further research and application of LLMs in domains that rely heavily on predictive modeling.
The researchers have demonstrated that the models trained on large, diverse datasets are adept at generalizing, making them particularly useful for real-world applications where data can vary drastically in size and complexity.
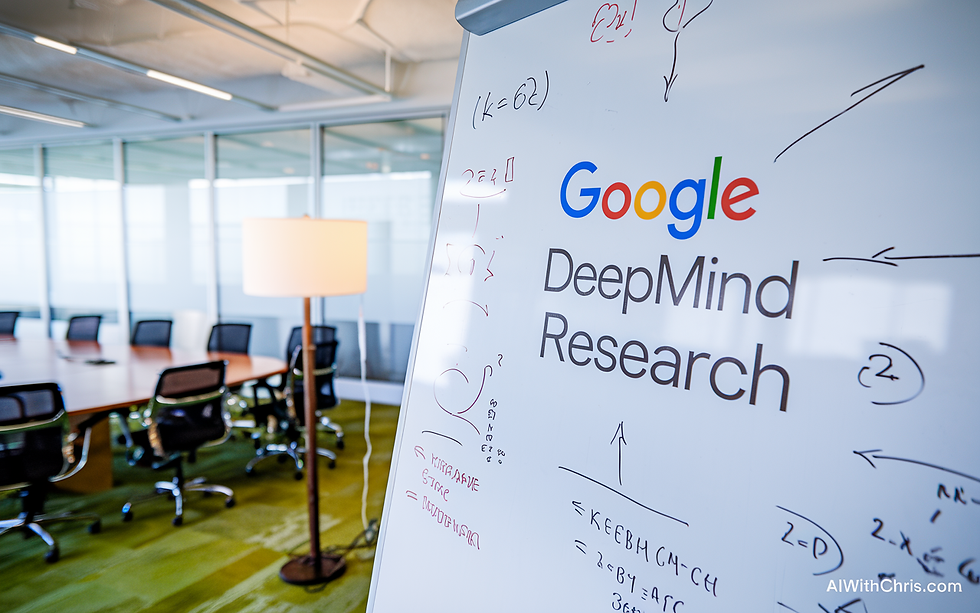
Transforming Features for Enhanced Accuracy and Speed
The ability of LLM embeddings to transform features is crucial for enhancing the accuracy and speed of regression analysis. By using these embeddings, data scientists have observed a significant reduction in prediction error while also increasing the computation speed of their models.
This is particularly advantageous in environments where quick decision-making is paramount, such as in financial markets or healthcare diagnostics.
The embeddings enable models to grasp the underlying patterns in the data without the need for exhaustive feature engineering, which can be both time-consuming and complex. Consequently, organizations can allocate resources more effectively, focusing on higher-impact tasks rather than getting mired in data manipulation.
Real-World Applications of LLM-Enhanced Regression Models
LLM-enhanced regression models have a plethora of applications across various sectors. In healthcare, for example, these models can predict patient outcomes based on a multitude of variables, such as genetic information, lifestyle choices, and environmental factors. In finance, businesses can leverage these models to forecast stock prices, optimizing their investment strategies.
Additionally, sectors like marketing can utilize LLM embeddings to analyze customer behaviors and preferences, allowing for more tailored advertising and product recommendations. As organizations increasingly require data-driven decisions, the ability to incorporate LLM embeddings into their regression analyses will likely become a game changer in unlocking insights previously thought unattainable.
The Future of LLM Embeddings in Predictive Analytics
Looking ahead, the potential of LLM embeddings in predictive analytics is vast. As research continues to advance, we can expect even more sophisticated models to emerge, further enhancing their effectiveness in regression tasks.
Researchers are continuously exploring the scalability and applicability of these models in diverse fields, leading to the development of new methodologies and best practices. Additionally, as computational power increases and data availability expands, the future of LLM embeddings seems more promising than ever.
We are likely to witness innovations that will allow these techniques to be more accessible, democratizing their application and enabling organizations of all sizes to leverage the power of predictive modeling.
Conclusion
Google DeepMind's research into LLM embeddings has opened new avenues for advanced regression analysis, showcasing their ability to enhance predictive capabilities across a variety of sectors. As organizations increasingly rely on data-driven insights, the integration of LLM embeddings into regression frameworks will become essential. This transformational approach not only improves accuracy and efficiency but also empowers teams to focus on strategic objectives rather than data management. The future of predictive analytics looks bright with LLMs paving the way for deeper insights and innovative applications in everyday decision-making.
Comments