Let's Master AI Together!
Detecting Fake Reviews Using Sentiment Analysis
Written by: Chris Porter / AIwithChris
How Sentiment Analysis Is Transforming the Fight Against Fake Reviews
The digital marketplace thrives on consumer reviews, functioning as a barometer for product quality and brand reputation. However, the increasing prevalence of fake reviews undermines this essential feature. The critical reality is that a significant portion of reviews on platforms like Amazon, Yelp, and Google are deceptive. As businesses grapple with maintaining authenticity, leveraging sentiment analysis emerges as a compelling solution. By analyzing consumer sentiments expressed in reviews, companies can pinpoint and mitigate the influence of fraudulent feedback.
Sentiment analysis, or opinion mining, is a branch of natural language processing that identifies and categorizes opinions occurring in a piece of text. The technology can detect polarity, meaning whether a review is positive, negative, or neutral. The significance of detecting fake reviews cannot be overstated. Fake reviews can distort consumer perception, misrepresent product quality, and unfairly harm genuinely reputable businesses. In a world rife with social proof, fake reviews dilute the capacity of honest voices to be heard.
So, how exactly does sentiment analysis contribute to detecting counterfeit reviews? The process typically involves using algorithms trained on previous review data, guiding the software in identifying inconsistencies. Language patterns, emotional cues, word frequency, and review length can signal potential fakes. For instance, a flurry of overly enthusiastic wording or a jagged review structure may indicate artificial inflation of ratings. More sophisticated algorithms leverage machine learning to implement advanced checks and balances, enhancing the speed and accuracy of detection.
Through real-time evaluation of reviews, sentiment analysis can flag potential fraud, giving businesses a chance to investigate further. By utilizing this tool effectively, companies can safeguard their reputational integrity while fostering a more transparent marketplace for consumers.
The Mechanisms and Benefits of Sentiment Analysis in Review Evaluation
Implementing sentiment analysis for detecting fake reviews employs various mechanisms and technologies. The backbone typically involves natural language processing (NLP) and machine learning algorithms. Each algorithm has different capabilities, such as supervised and unsupervised learning. Supervised learning involves training a model on labeled datasets of genuine and fake reviews, allowing the algorithm to learn the subtle intricacies that differentiate the two. Meanwhile, unsupervised learning can discover hidden patterns in an unlabeled dataset, helping to identify anomalies in reviews that may indicate fraud.
Along with algorithms, linguistic features play a pivotal role in evaluating reviews. Common indicators of fake reviews may include spammy language, excessive use of superlatives, a lack of specific details, or the repetition of certain phrases. Identifying these linguistic cues can significantly boost the detection rate of fake reviews. For example, an overly generic review that lacks authentic storytelling may signal a manipulated feedback. A robust sentiment analysis tool can draw from vast datasets to learn and adapt to these indicators over time.
The benefits of employing sentiment analysis for fake review detection extend beyond just identification. Businesses can also enhance their overall reputation monitoring strategies. By actively managing and improving their products or services based on genuine feedback, they can foster improved customer relationships. Moreover, consistent use of sentiment analysis promotes transparency, which can encourage loyal customers who place trust in the brand's integrity.
Engaging in active review monitoring can also preemptively address potential negative fallout from deceptive reviews. By responding to flagged reviews—whether fake or legitimate—companies can demonstrate their commitment to quality and accountability. This proactive approach not only helps identify potential misconduct but also lets customers know that their voices matter, thereby enhancing brand loyalty.
Only put the conclusion at the bottom of this content section.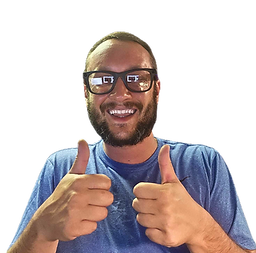_edited.png)
🔥 Ready to dive into AI and automation? Start learning today at AIwithChris.com! 🚀Join my community for FREE and get access to exclusive AI tools and learning modules – let's unlock the power of AI together!